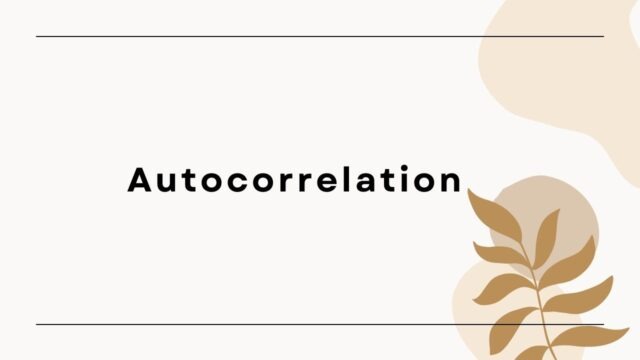
What is autocorrelation and what are its uses
Autocorrelation is a statistical measure that assesses the degree to which a time series is correlated with itself at different time lags. It is used to identify and quantify potential patterns in time series data. Autocorrelation can be used to detect seasonality in data, as well as to identify and quantify relationships between different time series.
Autocorrelation is often used in financial time series analysis to help identify price patterns and predict future movements. It can also be used in other areas such as studying population dynamics or analyzing environmental data.
Autocorrelation is calculated using the Pearson correlation coefficient, which measures the linear relationship between two variables. The autocorrelation of a time series at lag k is simply the Pearson correlation coefficient between the time series and itself at lag k.
How to calculate autocorrelation
To calculate autocorrelation, you will need to use the Pearson correlation coefficient. This measures the linear relationship between two variables. The autocorrelation of a time series at lag k is simply the Pearson correlation coefficient between the time series and itself at lag k.
To calculate the autocorrelation of a time series, you will need to:
- Choose a lag k (this is the number of time intervals that you want to shift the time series by)
- Calculate the Pearson correlation coefficient between the time series and itself at lag k
- Repeat this for different values of k to get a range of autocorrelation coefficients
Types of autocorrelation
There are three types of autocorrelation: positive, negative, and zero.
Positive autocorrelation occurs when a time series is more correlated with itself at higher lags than at lower lags. This indicates that there is a trend in the data, with the past values tending to be more similar to the current value than to previous values.
Negative autocorrelation occurs when a time series is more correlated with itself at lower lags than at higher lags. This indicates that there is a trend in the data, with the past values tending to be more similar to previous values than to the current value.
Zero autocorrelation occurs when a time series is equally correlated with itself at all lags. This indicates that there is no trend in the data and that it is behaving randomly.
It is important to note that not all time series will exhibit one of these three types of autocorrelation. Some may have positive autocorrelation at some lags and negative autocorrelation at others, while still others may have no autocorrelation at all.
Interpretation of autocorrelation coefficients
The autocorrelation coefficients are used to quantitatively measure this relationship. The interpretation of these coefficients can be quite complex, but there are some general guidelines that can be followed. First, the size of the coefficient indicates the strength of the correlation. Second, the sign of the coefficient indicates the direction of the correlation.
Positive autocorrelation means that the signal is correlated with itself at positive lag values (i.e., it is delayed with respect to itself), while negative autocorrelation means that the signal is correlated with itself at negative lag values (i.e., it leads itself). Finally, the significance of the coefficient indicates whether or not the correlation is statistically significant. In general, autocorrelation coefficients close to 1 or -1 are considered to be highly significant, while coefficients close to 0 are not considered to be significant.