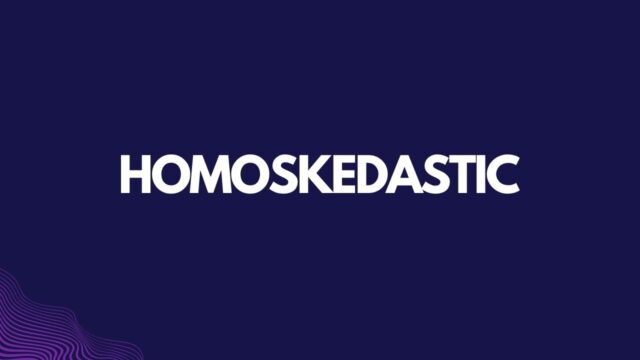
What is homoskedasticity and why is it important in statistics
In statistics, homoskedastic is the assumption that the variance of a dependent variable is constant across all values of the independent variable. In other words, homoskedasticity means that the predictors do not have a systematic impact on the outcome variable. This assumption is important because it allows statisticians to make assumptions about the distribution of errors, which in turn allows them to make better predictions. Without homoskedasticity, these predictions would be less reliable.
Although homoskedasticity is a strong assumption, it is often met in practice. When it is not met, statisticians can use special techniques to account for the non-constant variance. These techniques help to ensure that statistical predictions are as accurate as possible.
How to test for homoskedasticity in your data
Testing for homoskedasticity is important because it can affect the interpretation of your results. A significant amount of heterogeneity in the error term can lead to problems like omitted variable bias and incorrect standard errors. There are a f
The most common method is to use a Chow test, which compares the variances of two groups of data points. You can also use graphical methods like residual plots or scatterplots to detect heteroskedasticity. If you suspect that your data is heteroskedastic, you can use transformation techniques like logarithmic transformation to try to reduce the amount of variability in the dependent variable.
The benefits of knowing whether your data is homoskedastic
There are several benefits to knowing whether your data is homoskedastic. First, it can help you to avoid making inaccurate conclusions about your data. Second, it can help you to choose the most appropriate statistical methods for analyzing your data. Third, it can help you to better understand the relationships between variables. Finally, it can help you to detect outliers and other unusual patterns in your data. Thus, understanding homoskedasticity can be extremely helpful in a variety of settings.
How to adjust for heteroskedasticity in your analyses
There are a number of ways to adjust for heteroskedasticity, including transforming the data, using weighted least squares regression, and computing robust standard errors. Each of these methods has its own advantages and disadvantages, so it is important to choose the one that is most appropriate for your data and your goals. With careful planning and execution, heteroskedasticity can be successfully addressed, and accurate results can be obtained.
Examples of when homoskedastic is violated in real-world data
There are a number of real-world examples of when homoskedasticity is violated. For instance, earnings data is often heteroskedastic, with executives and professionals earning much higher incomes than the rest of the population. This can lead to problems when using ordinary least squares regression to analyze earnings data, as the results may be biased.
Another example comes from environmental science, where it is common for pollution levels to be higher in urban areas than in rural areas. This violates the assumption of homoskedasticity, as the variance of pollution levels is not constant across all regions. These examples illustrate how violating the assumption of homoskedasticity can lead to problems when analyzing data.
Ways to improve your analyses when homoskedasticity is not met
When homoskedasticity is not met, there are a few different ways you can improve your analyses. One way is to use weighted least squares regression, which assigns different weights to each observation. This can be done by using the inverse of the variance of each observation as the weight. Another way to improve your analyses is to use robust standard errors. This technique downweights outliers, which can help to reduce the influence of heteroskedasticity on your results.
Finally, you can also transform your data so that it meets the assumptions of homoskedasticity. This can be done by taking the natural log of your dependent variable, which can help to stabilize the variance. By using these techniques, you can improve your results even when heteroskedasticity is present.