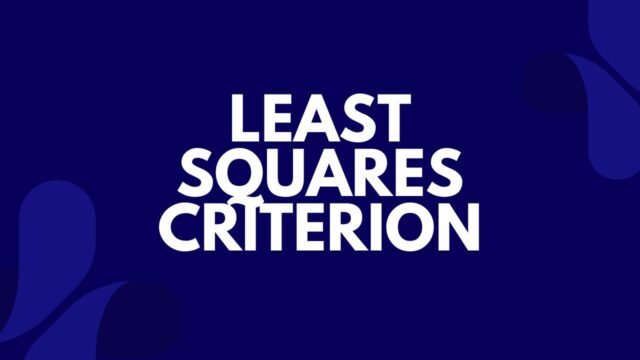
What is the least squares criterion
The least squares criterion is a statistical technique used to find the best fit for a set of data points. The technique minimizes the sum of the squared errors between the data points and the line of best fit. The least squares criterion is often used in linear regression, where it can be used to find the slope and intercept of the line of best fit. The technique can also be used in nonlinear regression, where it can be used to find the best fit for a curve. In general, the least squares criterion provides a way to find the line or curve that best fits a set of data points.
How does the least squares criterion work
The least squares criterion is a statistical method used to estimate the parameters of a linear regression model. The technique is called “least squares” because it minimizes the sum of the squared residuals, or prediction error. In other words, it finds the line of best fit for a set of data points. The least squares criterion is used in many different fields, from economics to engineering.
In each case, the goal is to find the line (or curve) that best fits the data. This line can then be used to make predictions about future values. The advantage of the least squares criterion is that it is relatively easy to compute and understand. The disadvantage is that it can be sensitive to outliers, or data points that are far from the rest of the data. When outliers are present, the least squares criterion may not produce the best possible results.
How can you use the least squares criterion to solve problems
The least squares criterion is a mathematical tool that can be used to solve problems in a variety of fields, from engineering to economics. The principle behind the least squares criterion is simple: when multiple variables are being considered, the one that minimizes the sum of the squared deviations from the mean is the best fit. This approach can be used to find the line of best fit for a set of data points, or to estimate unknown values based on known data. In many cases, the least squares criterion provides a more accurate solution than other methods, making it an essential tool for anyone working with large data sets. Thanks to the versatility of the least squares criterion, it is sure to remain a valuable tool for problem-solving in the years to come.
What are some of the benefits of using the least squares criterion
There are many benefits to using the least squares criterion, including its ability to handle large amounts of data and its relatively simple mathematical implementation. Additionally, the least squares criterion is very robust, meaning that it produces accurate results even when there are outliers in the data. Overall, the least squares criterion is a powerful statistical tool that can be used to great effect in a variety of situations.
What are some of the drawbacks of using the least squares criterion
The least squares criterion is a statistical method used to estimate the parameters of a linear regression model. This criterion minimizes the sum of the squared residuals, which are the differences between the observed values and the predicted values. While the least squares criterion is widely used, it has some potential drawbacks. One issue is that it is heavily influenced by outliers, which can distort the results. Additionally, the least squares criterion assumes that the errors are normally distributed, which may not be the case in real-world data sets. Finally, this criterion does not account for certain types of dependencies among the variables, which can lead to inaccurate results. Despite these drawbacks, the least squares criterion remains a popular tool for statistical analysis.